Deep Neural Labeling: Hybrid Hand Pose Estimation Using Unlabeled Motion Capture Data With Color Gloves in Context of German Sign Language
Kristoffer Waldow, Arnulph Fuhrmann, Daniel Roth
In: 6th IEEE International Conference on Artificial Intelligence & extended and Virtual Reality 2024 (AIxVR ’24)
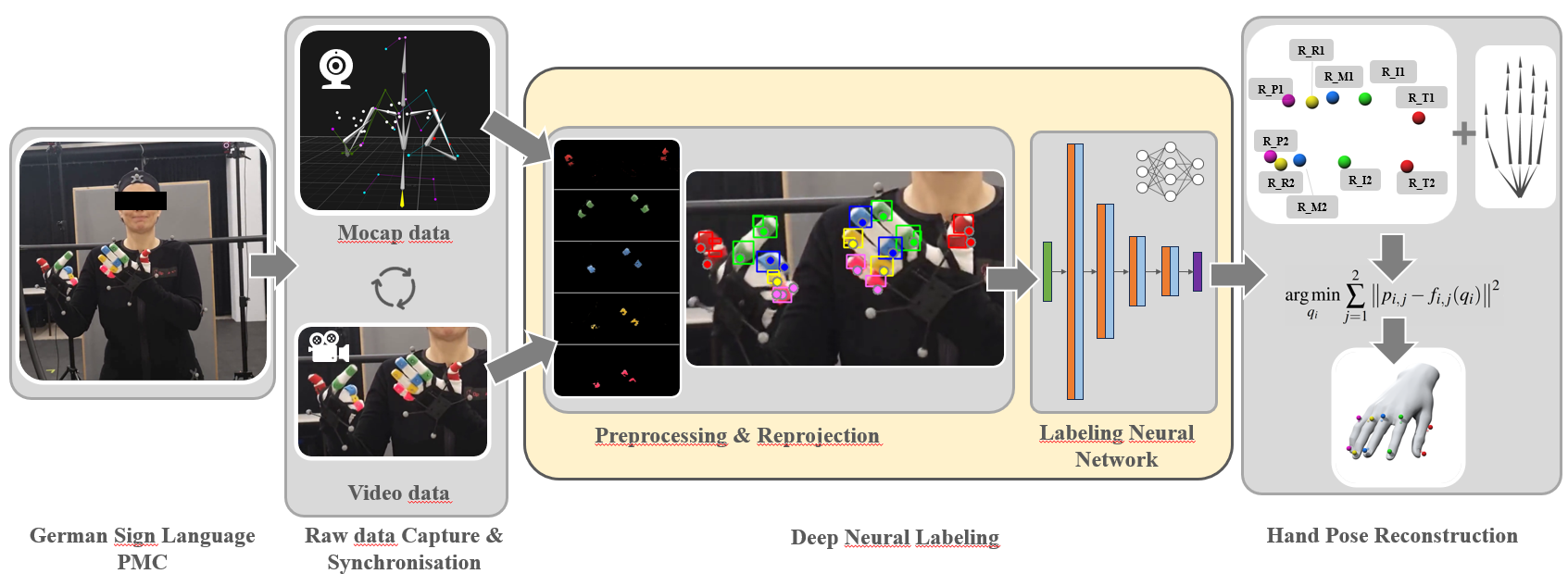
Abstract:
Hands are fundamental to conveying emotions and ideas, especially in sign language. In the context of virtual reality, motion capture is becoming essential for mapping real human movements to avatars in immersive environments. While current hand motion capture methods feature partly great usability, accuracy, and real-time performance, they have limitations. Industry-standard motion capture methods with sensor gloves lead to acceptable results, but still produce occasional errors due to proximity of the fingers and sensor drifts. This, in turn, requires time-consuming correction and manual labeling of optical markers during post-processing for offline use cases and prohibits the use in real-time scenarios as VR communication. To overcome these limitations, we introduce a novel hybrid hand pose estimation method that leverages both an optical motion capture system and a color-coded fabric glove. This approach merges the strengths of both techniques, enabling the automated labeling of 3D marker positions through a data-driven machine-learning approach. Using a spherical capture rig and a deep learning algorithm, we improve efficiency and accuracy. The labeled markers then drive a robust optimization procedure for solving hand posture, accounting for limitations in finger movements and validation checks. We evaluate our system in the context of German sign language where we achieve an accuracy of 97% correct marker assignments. Our approach aims to enhance the accuracy and immersion of sign language communication in VR, making it more inclusive for both deaf and hearing people.